This National Science Foundation EPSCoR grant (#2420405) will support research and education activities to generate new knowledge about the interplay between climate change and agriculture. It will focus on promoting the use of sensor networks, data analyses, artificial intelligence, and social studies in solving engineering and science problems.
Agriculture-based communities in the central region of the United States are disproportionally affected by dynamic weather and climate change, yet they often face challenges in real-time weather prediction, precise climate assessment, and climate resilience due to a lack of sufficient weather stations.
To address these challenges, this project aims to design and implement the Data-Advanced Research and Education (DARE) infrastructure. This will enhance weather and soil data collection, improve climate forecasting, and bolster workforce education, ultimately increasing the climate resilience of ag-based communities.
This project is a collaboration among universities in four NSF EPSCoR jurisdictions: the University of Iowa, the University of Arkansas, Kansas State University, and the University of Nebraska-Lincoln. Through this research, the project team plans to establish a citizen network for real-time, high-resolution data collection and develop AI-assisted models for accurate weather prediction.
Additionally, they will explore environmental fairness issues to help ag-based communities better withstand climate change. The project will also focus on workforce development and education by engaging citizens, including K-12 students, through summer camps, field campaigns, and statewide ag-extension offices. It will involve community health workers, grower organizations, STEM education offices, and the DARE Virtual service system. Particular emphasis will be placed on educating, mentoring, and training undergraduate and graduate students, post-doctoral scholars, early career faculty, and community members such as farmers and industry personnel.
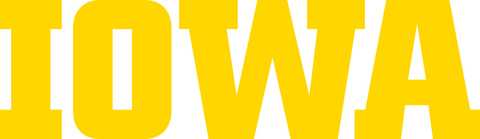
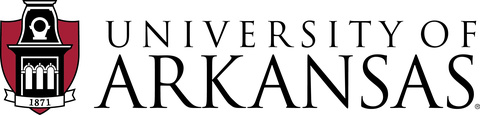
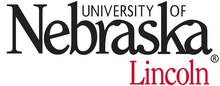

University of Iowa
| EPSCoR RII-FEC |

This material is based upon work supported by the National Science Foundation under Grant Number 2420405. Any opinions, findings, and conclusions or recommendations expressed in this material are those of the author(s) and do not necessarily reflect the views of the National Science Foundation.